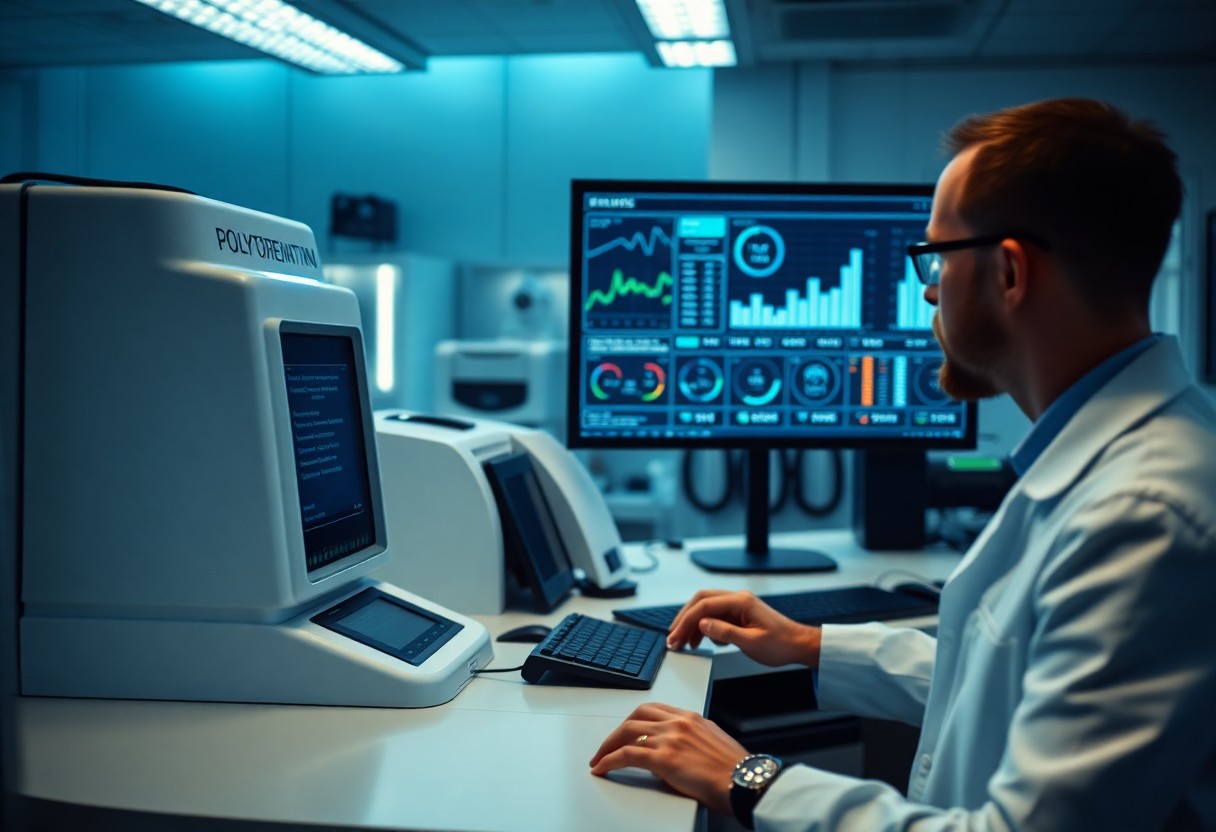
It’s important to understand how data analytics can enhance your approach to polygraph science. By leveraging data-driven insights, you can improve the accuracy and reliability of lie detection methodologies. This guide provides you with strategies to analyze patterns in physiological responses, assess test results more effectively, and optimize questioning techniques. Embracing data analytics not only enhances your skills but also fosters confidence in your results. Get ready to elevate your polygraph practice to the next level!
Key Takeaways:
- Data analytics can enhance the accuracy of polygraph results by providing insights into patterns and correlations in physiological responses during testing.
- Implementing machine learning algorithms allows for the continuous improvement of polygraph techniques, enabling more reliable identification of deceptive behavior.
- Collaboration between data scientists and polygraph experts is crucial for developing comprehensive models that integrate psychological factors and contextual variables influencing test outcomes.
1. Utilize statistical models for enhanced polygraph accuracy.
2. Integrate machine learning for predictive analysis.
3. Analyze historical data for trend identification.
4. Implement real-time monitoring for immediate insights.
5. Combine diverse data sources for comprehensive evaluations.
6. Regularly update methodologies based on analytical findings.
Understanding Polygraph Fundamentals
While engaging with the science of polygraphy, it’s necessary to grasp the foundational elements that drive its functionality. Understanding how a polygraph operates will equip you with the necessary knowledge to research deeper into data analytics applications that can enhance the accuracy and reliability of lie detection methods.
Traditional Polygraph Components
Fundamentals of a traditional polygraph include three vital components: a respiration sensor, galvanic skin response, and a cardiovascular monitor. These instruments work in tandem to record physiological changes during questioning, providing insights into the subject’s emotional state, which serves as a basis for interpreting truthfulness.
Physiological Response Measurements
Among the fundamental aspects of a polygraph are the physiological response measurements that it captures. These measurements are vital indicators of your emotional reactions during interrogation.
Response measurements are based on your heart rate, blood pressure, and skin conductance, which together provide significant insights into your anxiety levels or stress that may be linked to deception. The physiological response is considered a strong indicator; an increase in these measurements often suggests discomfort or untruthfulness. Understanding these parameters can aid in interpreting the results more effectively, allowing you to analyze not just the data but the psychological aspects of the individual undergoing testing.
Data Analytics in Modern Polygraph Science
Even as technology advances, integrating LIE DETECTION GUIDE principles into data analytics enhances your understanding of polygraph outcomes. By using sophisticated data analytics, you can refine the methodologies behind polygraph testing, improving accuracy and reliability in lie detection. This transformation allows for critical insights that help professionals respond to evolving challenges in the ever-complex field of behavioral assessment.
Machine Learning Applications
Analytics in polygraph science empowers you to utilize machine learning techniques, which can identify patterns in physiological data that humans may overlook. These advanced algorithms analyze vast datasets, adjusting their predictive capabilities as more information becomes available, ultimately leading to enhanced precision in lie detection.
Pattern Recognition Algorithms
Polygraph testing greatly benefits from the implementation of pattern recognition algorithms which meticulously analyze physiological responses to determine deception cues. By employing these algorithms, you can assess variables such as heart rate, respiration, and galvanic skin response more effectively than traditional methods.
In addition, employing pattern recognition algorithms in polygraph science enhances your ability to detect subtle variations in physiological responses that may indicate deception. These algorithms can uncover trends that are often difficult to observe with the naked eye, providing a more data-driven approach to lie detection. The reliance on machine learning aids in continuously improving the model’s capability as it learns from new data, ultimately increasing the accuracy of results. As you harness these advanced techniques, you enhance both the credibility and reliability of polygraph evaluations.
Data Collection and Preprocessing
All effective data analysis begins with robust data collection and preprocessing. Accurate and reliable data is vital in refining polygraph science, as it forms the foundation for further analysis. For an insightful examination of this field, refer to A review of the polygraph: history, methodology and … which discusses methodologies pertinent to polygraph data handling.
Sensor Data Quality Management
Along your data collection journey, ensuring sensor data quality is paramount. You must implement consistent calibration and maintenance checks for all sensors used in polygraph testing. This attention to quality not only enhances data accuracy but also boosts the reliability of your analytical outcomes.
Signal Processing Techniques
Sensor data is often noisy and requires robust processing techniques to extract valuable insights. The adoption of effective signal processing techniques can significantly improve the analysis of polygraph data. Techniques such as filtering, Fourier transforms, and wavelet analysis allow you to isolate relevant signals from background noise. This processing is vital for accurate interpretations of physiological responses during polygraph examinations, enhancing the overall reliability of the results. Properly applying these techniques ensures that you can make informed decisions based on the most pertinent data from your tests.
Statistical Analysis Methods
Many statistical analysis methods play a vital role in refining polygraph science by providing insights into the underlying patterns and relationships within the data. By employing various techniques such as regression analysis, factor analysis, and hypothesis testing, you can identify significant factors affecting the reliability and accuracy of polygraph results. This analytical approach not only enhances the overall credibility of polygraph examinations but also aids in establishing empirical guidelines for test administration and interpretation.
Multivariate Analysis
Around polygraph data, multivariate analysis allows you to assess multiple variables simultaneously, giving you a comprehensive view of how different factors interact with each other. By using techniques such as principal component analysis or CANONICAL correlation, you can uncover hidden patterns within the data that may influence the outcome of polygraph results.
Time Series Analysis
Along with exploring relationships between variables, time series analysis enables you to examine data points collected over time, helping to identify trends, seasonal patterns, and cycles in polygraph outcomes. This analysis provides valuable insights into how physiological responses may change under varying conditions and over different periods.
Another important aspect of time series analysis is its ability to enhance your predictive capabilities. By understanding historical patterns, you can make informed predictions about future behaviors or responses, which can be particularly valuable in assessing the potential validity of polygraph test results. Identifying trends in physiological data can also inform adjustments to scoring algorithms, thereby improving the overall accuracy of polygraph findings. Additionally, be aware that seasonal variations may influence results, so incorporating this dimension into your analysis can lead to a more nuanced understanding of the data.
Advanced Analytics Integration
Unlike traditional methods, integrating advanced analytics into polygraph science allows you to gain deeper insights and enhance accuracy. This approach revolutionizes how data is interpreted and utilized. Consider the following benefits:
- Enhanced data visualization
- Predictive analytics for trend identification
- Improved decision-making processes
- Increased efficiency in data handling
Analytics Breakdown
Aspect | Description |
---|---|
Data Collection | Aggregating diverse data sources |
Data Analysis | Employing statistical tools for interpretation |
Outcome Evaluation | Assessing the effectiveness of decisions |
Artificial Intelligence Implementation
With AI’s integration, you can optimize polygraph technology by leveraging algorithms that analyze behavioral patterns, significantly enhancing accuracy. AI models can assess indicators of deception more effectively than conventional methods, leading to improved testing outcomes.
Real-time Data Processing
At the core of modern polygraph science, real-time data processing empowers you to react promptly to changes during examinations, enhancing the accuracy of results.
Understanding real-time data processing means being able to analyze physiological responses as they happen, allowing you to adjust questioning strategies instantly. This capability can significantly reduce the chances of false positives and negatives in results. By leveraging real-time data, you can identify crucial patterns that indicate deception or truthfulness, leading to more informed decisions. Having immediate insights enables you to maintain the integrity of the examination while providing a more dynamic approach to understanding physiological reactions during testing.
Quality Assurance and Validation
For effective polygraph science, implementing rigorous quality assurance and validation protocols is vital. This ensures the accuracy and reliability of your data analysis processes. By establishing clear guidelines and standards, you can consistently assess the performance of your polygraph instruments and methodologies. Additionally, maintaining thorough documentation will support accountability and foster an environment of continuous improvement in your analytical practices.
Cross-validation Techniques
Beside ensuring accuracy, cross-validation techniques help you evaluate the predictive power of your polygraph data analysis models. By dividing your dataset into subsets, you can train your model on one subset and test it on another, providing a robust assessment of its performance. This method helps in identifying any overfitting issues and enhancing the generalization of your models.
Error Rate Assessment
Below, understanding and calculating error rates are vital to gauge the effectiveness of your polygraph analysis. Performing regular assessments allows you to identify patterns of false positives or negatives, which can severely impact the credibility of your findings.
Another important aspect of error rate assessment is the identification of specific error types, such as false positives, where you incorrectly indicate deception, and false negatives, where truthful responses are misclassified as deceptive. Analyzing these errors enables you to refine your methodologies, thereby improving the overall accuracy of your results. Furthermore, minimizing error rates not only enhances the reliability of polygraph outcomes but also supports the ethical use of this technology in various applications, reinforcing its value in decision-making processes.
Summing up
On the whole, utilizing data analytics to refine polygraph science empowers you to enhance the accuracy and reliability of lie detection. By systematically analyzing vast datasets, you can identify patterns, integrate advanced algorithms, and continuously improve your methodologies. This comprehensive approach not only strengthens your understanding of physiological responses but also enhances your decision-making processes. Embracing data-driven techniques allows you to remain at the forefront of your field, ultimately leading to more effective polygraph assessments.
FAQ
Q: How can data analytics improve the accuracy of polygraph results?
A: Utilizing data analytics can significantly enhance the accuracy of polygraph results by enabling a deeper analysis of physiological responses. By integrating large datasets collected from various polygraph tests, data analytics techniques can identify patterns and correlations that may not be immediately apparent. This allows for more precise interpretations of the data, as algorithms can help differentiate between truthful and deceptive responses with higher sensitivity and specificity. Additionally, predictive modeling can be applied to assess the likelihood of truthfulness based on historical data, further refining the assessment process.
Q: What types of data should be collected for effective analysis in polygraph studies?
A: For effective analysis in polygraph studies, a variety of data types should be collected, including physiological metrics (such as heart rate, blood pressure, and respiration), demographic information of the subjects (age, gender, psychological profiles), and contextual variables (the nature of the questions asked, the setting of the test, etc.). Collecting both qualitative and quantitative data allows for comprehensive analysis. It is also beneficial to gather data from previous tests to identify trends and improve predictive accuracy. Ensuring that the data is well-cleaned and structured is necessary for any subsequent analytical processes.
Q: How can practitioners implement data analytics in their polygraph examinations?
A: Practitioners can implement data analytics in their polygraph examinations by starting with the collection of expansive datasets from their tests. They should familiarize themselves with analytical tools and software that are designed for psychological and physiological analysis. Collaborating with data analysts or data scientists can also enhance the understanding of complex algorithms and statistical methods. Conducting workshops or training sessions focusing on data interpretation will further empower practitioners to interpret results more effectively. Finally, incorporating machine learning algorithms can help automate and streamline the analysis process, allowing for faster and more accurate evaluations.