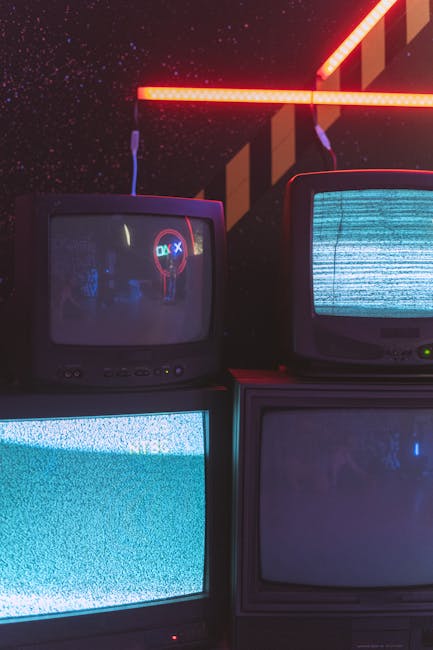
Ethical Challenges and Solutions in Deception Detection
In an era where information is abundant and easily accessible, deception detection has become an essential tool in various fields ranging from law enforcement to business negotiations. The ability to accurately detect deceit can have significant consequences, including safeguarding national security, ensuring justice, and maintaining corporate integrity. However, the process of deception detection is fraught with ethical challenges. This blog post explores these ethical dilemmas and offers actionable solutions to address them.
Introduction
Deception detection involves identifying when an individual is not being truthful. Techniques range from traditional methods such as behavioral analysis and polygraph tests to advanced technologies like artificial intelligence (AI) and facial recognition. While these methods can be effective, they also raise ethical concerns. How do we balance the need for truth with respect for individual rights? What measures can be implemented to ensure ethical practices in deception detection?
The Importance of Deception Detection
Deception detection plays a crucial role in various sectors:
- Law Enforcement: Identifying deceit can be critical in criminal investigations.
- Corporate World: Ensuring honesty in financial reporting and internal communications.
- National Security: Detecting potential threats through intelligence gathering.
Despite its importance, deception detection is not without ethical challenges. These challenges must be addressed to ensure that the practice is both effective and morally sound.
Ethical Challenges in Deception Detection
Privacy Concerns
One of the primary ethical challenges is the invasion of privacy. Techniques like polygraph tests and AI-based facial recognition systems can be intrusive, often requiring individuals to disclose personal information or undergo detailed scrutiny. According to a survey conducted by Pew Research Center, 77% of Americans believe that their personal data is less secure than it was five years ago. This growing concern about privacy is exacerbated by the use of invasive deception detection methods.
Accuracy and Reliability
The accuracy of deception detection methods is another significant ethical concern. False positives (identifying truthful individuals as deceptive) and false negatives (failing to detect deceit) can have severe consequences. For example, a false positive in a criminal investigation could lead to wrongful convictions, while a false negative could allow a guilty party to evade justice. Studies have shown that traditional polygraph tests have an accuracy rate of about 70-90%, but this is not foolproof.
Bias and Discrimination
Bias in deception detection is a critical issue, particularly with AI-based systems. Algorithms can inadvertently incorporate biases present in the training data, leading to discriminatory practices. For instance, facial recognition technology has been found to have higher error rates for people of color. A study by the National Institute of Standards and Technology (NIST) revealed that facial recognition algorithms are less accurate in identifying individuals from minority groups, raising ethical concerns about fairness and equality.
Informed Consent
Obtaining informed consent is essential but challenging in deception detection. Individuals must be fully aware of the methods being used, the data being collected, and the potential consequences. However, in many cases, individuals may feel coerced into consenting, particularly in situations involving power imbalances, such as employer-employee relationships or law enforcement interrogations.
Solutions to Ethical Challenges in Deception Detection
Enhancing Privacy Protections
To address privacy concerns, organizations can implement robust data protection measures. This includes anonymizing data, using encryption, and limiting access to sensitive information. Additionally, transparency about the methods used and the data collected can help build trust and alleviate privacy concerns.
Improving Accuracy and Reliability
Investing in research and development to enhance the accuracy of deception detection methods is crucial. This includes refining algorithms, incorporating diverse datasets, and continually validating the effectiveness of these methods. Organizations can also employ a combination of techniques to cross-verify results, thereby reducing the risk of false positives and negatives.
Addressing Bias and Ensuring Fairness
To mitigate bias, it is essential to use diverse and representative datasets in training AI algorithms. Regular audits and assessments can help identify and rectify biases. Additionally, involving ethicists and diverse stakeholders in the development and implementation of deception detection systems can ensure a more equitable approach.
Ensuring Informed Consent
Organizations must prioritize obtaining genuine informed consent. This involves providing clear and comprehensive information about the methods, data collection, and potential implications. Additionally, individuals should have the right to opt-out without facing adverse consequences. Establishing independent oversight bodies can help ensure that consent is obtained ethically and transparently.
Case Studies and Examples
Case Study: Polygraph Tests in Law Enforcement
Polygraph tests have long been used in law enforcement to detect deceit. However, their reliability and ethical implications have been a subject of debate. In 2003, the U.S. Department of Energy conducted a study on the accuracy of polygraph tests, revealing an accuracy rate of approximately 85%. Despite this, the potential for false positives and the intrusive nature of the tests have led to calls for alternative methods.
Example: AI in Corporate Hiring
Many corporations have started using AI-based tools to detect deceit during hiring processes. While these tools can help identify dishonest candidates, they also raise ethical concerns. For instance, in 2018, Amazon scrapped an AI hiring tool after discovering that it was biased against women. This example highlights the importance of addressing bias and ensuring fairness in AI-based deception detection.
Conclusion
Deception detection is an invaluable tool in various sectors, from law enforcement to corporate settings. However, the ethical challenges associated with these methods cannot be overlooked. By enhancing privacy protections, improving accuracy, addressing bias, and ensuring informed consent, organizations can navigate these ethical dilemmas effectively. As technology continues to evolve, it is crucial to remain vigilant and committed to ethical practices in deception detection.
Ultimately, the goal is to balance the need for truth with respect for individual rights, ensuring that deception detection serves as a force for good rather than a source of harm. By implementing the solutions outlined in this blog post, organizations can achieve this balance and foster a more ethical approach to deception detection.