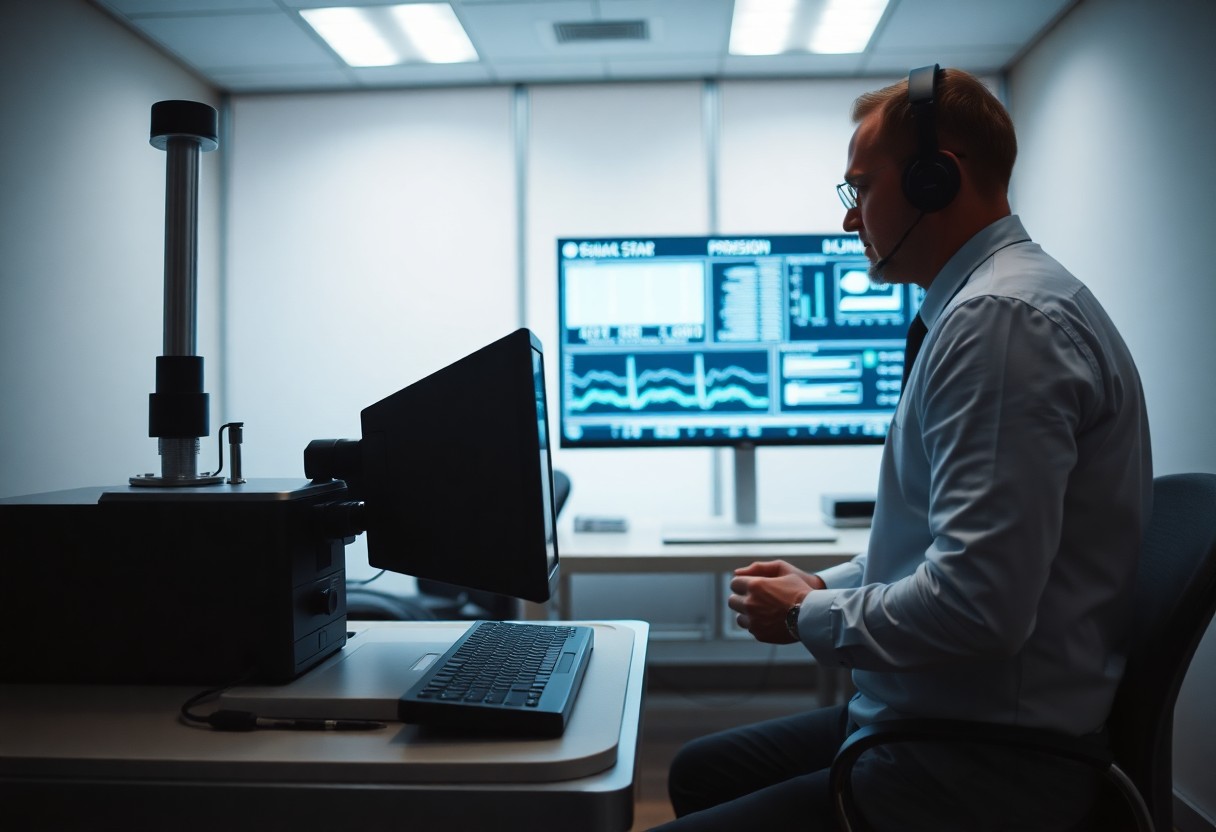
NLP can transform your approach to polygraph examinations by enhancing the accuracy of verbal statements analysis. By incorporating advanced algorithms, you can detect deceptive behaviors more effectively, leveraging semantic understanding to interpret emotional indicators within testimonies. This integration not only improves the overall efficiency of your examinations but also lends a scientific basis to your findings, making your assessments more reliable. In this post, you will discover practical steps to implement NLP techniques, enabling you to elevate your polygraph practice.
Key Takeaways:
- Natural Language Processing (NLP) can enhance the accuracy of polygraph interpretations by analyzing verbal responses for stress indicators and inconsistencies.
- Integrating NLP tools can automate the detection of linguistic patterns, helping examiners focus on critical areas of questioning without missing subtle cues.
- Combining traditional polygraph techniques with NLP insights can lead to a more holistic approach, improving overall assessment and decision-making in investigations.
1. Utilize NLP to analyze language patterns in responses.
2. Enhance accuracy by identifying emotional cues in speech.
3. Implement machine learning to improve data interpretation.
4. Streamline analysis with automated language processing tools.
5. Train examiners on NLP technology for effective integration.
6. Combine traditional methods with NLP for comprehensive results.
Understanding Polygraph Fundamentals
For a comprehensive grasp of polygraph examinations, it’s vital to understand the principles driving these tests. A polygraph measures physiological responses—such as heart rate, blood pressure, and respiration—to assess truthfulness. The underlying assumption is that deceptive answers trigger heightened emotional responses, reflected through these bodily signals. By becoming familiar with the polygraph’s operation, you can appreciate the complexities of data interpretation and its implications for accurate assessments.
Traditional Polygraph Components
Around the world, traditional polygraphs consist of important components including sensors that monitor physiological functions, a recording device, and the examiner. These components work together to capture responses during questioning. The examiner plays a vital role in interpreting the data and forming conclusions based on the physiological changes observed, blending both technology and human insight.
Current Interpretation Methods
Behind modern polygraph examinations lies a mix of traditional techniques and emerging methodologies. As you navigate through these interpretations, you’ll discover that the reliance on examiner intuition and established scoring systems remains prevalent. However, there is a growing integration of data analytics to support human judgment, potentially enhancing your understanding of results.
A significant trend in current interpretation methods is the use of advanced computer algorithms and artificial intelligence, which can analyze vast datasets to identify patterns and correlations that might elude human examiners. By incorporating objective insights, these technologies aim to improve accuracy and reduce examiner bias. This evolution not only enhances your ability to interpret results but can also foster greater confidence in polygraph accuracy. Though opportunities for error exist, leveraging these advancements can lead to more reliable outcomes, ensuring that your assessments are grounded in both technology and expertise.
Natural Language Processing Basics
Assuming you are venturing into the integration of Natural Language Processing (NLP) in polygraph examinations, it’s crucial to start with the fundamentals. NLP is a branch of artificial intelligence that focuses on the interaction between computers and humans through natural language. By utilizing NLP, you can analyze and interpret verbal responses more effectively, aiding in the understanding of underlying emotions and truths during lie detection.
NLP Core Concepts
Beside the various applications NLP presents, having a solid grasp of its core concepts is necessary. These include syntax (structure of sentences), semantics (meaning of words and phrases), and pragmatics (contextual use of language). Understanding these principles enables you to utilize NLP tools more efficiently for extracting valuable insights from the responses given in polygraph exams.
Text Analysis Techniques
Against traditional methods, integrating NLP introduces advanced text analysis techniques that can significantly enhance the interpretative process in polygraph examinations. Techniques like sentiment analysis, tokenization, and named entity recognition allow you to dissect the language used by your subjects, uncovering valuable information that may not be immediately apparent.
For instance, sentiment analysis can reveal the emotional undertones of a subject’s words, helping you gauge their honesty. Tokenization breaks down sentences into individual components, making it easier for you to examine specific language patterns. Additionally, named entity recognition identifies key subjects or topics, providing contextual relevance that can aid in lie detection. By leveraging these text analysis techniques, you enhance your ability to interpret responses accurately, ultimately leading to more informed conclusions during examinations.
Integration Framework
Keep in mind that establishing an effective integration framework for incorporating Natural Language Processing (NLP) in polygraph examinations is crucial. This framework should align your NLP capabilities with the specific needs of polygraph testing, focusing on enhancing data interpretation through advanced analytics and linguistic analysis.
Data Collection Points
Integration of NLP begins with identifying key data collection points within your polygraph examination process. This includes the verbal exchanges during the test, written responses, and any relevant background information about the subject, allowing you to capture diverse linguistic patterns that can be analyzed for lie detection.
System Architecture Requirements
Any successful implementation of NLP technology requires a robust system architecture to support data processing and analysis. You should consider integrating scalable cloud solutions that facilitate real-time data input and analysis, ensuring quick response times while maintaining data integrity.
This architecture must also include secure data storage systems to protect sensitive information, as well as advanced algorithms capable of processing large datasets efficiently. Additionally, incorporating machine learning models will enhance your ability to detect patterns and anomalies in speech, improving the accuracy of your polygraph results. Ensuring that your system is capable of continuous learning from incoming data will ultimately lead to a more reliable analysis process.
Language Pattern Analysis
Unlike traditional methods of polygraph examination, integrating Natural Language Processing (NLP) allows for a deeper understanding of language patterns specific to individuals. This advanced technology analyzes not only the content of what is said but also the structure and nuances of language, giving you a richer perspective on the underlying messages conveyed during an examination. By recognizing unique speech patterns and variations, you can enhance your interpretative skills, leading to more reliable conclusions in polygraph assessments.
Verbal Response Processing
For effective verbal response processing, leveraging NLP techniques can aid in identifying emotional undertones and intentions behind spoken words. By using algorithms to assess tone, pacing, and word choice, you gain valuable insights into the subject’s mindset and potential motivations, thereby improving your evaluative accuracy. This data-driven approach helps pinpoint inconsistencies and enhances the overall quality of your analysis during polygraph examinations.
Deception Indicators in Speech
Between various verbal attributes, certain indicators may suggest deception, including speech hesitations, overly complex language, and avoidance of specific details. By analyzing these factors with NLP, you can discern subtle cues that reveal underlying dishonesty or unease while the subject speaks. This automated processing helps you focus on significant deviations from baseline behaviors established prior to the examination.
Language plays a pivotal role in uncovering deception indicators during polygraph examinations. Subtle elements such as repetitive phrasing, shifts in verbal tempo, and the frequency of filler words like “um” and “uh” can signal uneasiness or attempts to fabricate reality. By identifying these key traits, you enhance your ability to detect dishonesty and improve the reliability of your findings. Staying attuned to these indicators, especially in high-stakes situations, is imperative for ensuring competent and informed interpretation in your polygraph assessments.
Real-time Processing Implementation
Many practitioners are now embracing real-time processing methodologies in polygraph examinations. This approach allows for immediate analysis of physiological data, enhancing the examiner’s ability to interpret responses accurately. Integrating Neural Network Applications in Polygraph Scoring&mdash can significantly increase the efficiency and reliability of your findings.
Software Integration
About ensuring a seamless experience, effective software integration is key to harnessing the full potential of natural language processing and polygraph technologies. Utilize compatible platforms and APIs to collectively process the data, improving your analysis and reporting capabilities.
Hardware Requirements
For successful implementation, you need to be aware of the necessary hardware specifications. Quality sensors, robust computing devices, and reliable connectivity are vital components in acquiring accurate physiological data.
In addition, select high-quality sensors that can reliably monitor and record physiological responses for precise data capture. Adequate processing power is also necessary, as real-time analytics require fast computation to interpret the data instantly. Ensuring you have a reliable internet connection guarantees consistent data transmission, imperative for real-time applications. Prioritizing these hardware aspects will immensely enhance your polygraph examination process.
Validation and Quality Control
Your approach to integrating Natural Language Processing (NLP) in polygraph examinations must include stringent validation and quality control measures. Implementing these practices ensures that your results are reliable and that the interpretations made during the examination are grounded in scientific methodology. For a comprehensive overview, refer to A Journal of Science and Field Practice to enhance your knowledge on this subject.
Accuracy Metrics
Against the backdrop of evolving technological landscapes, establishing clear accuracy metrics is crucial for gauging the effectiveness of NLP applications in polygraph examinations. You should focus on key indicators such as precision, recall, and F1 score to assess the performance of your system, ensuring that the insights derived are both valid and actionable.
Cross-validation Procedures
Quality in your NLP integration extends beyond initial findings; it requires robust cross-validation procedures.
At every stage of analysis, you must implement cross-validation techniques to enhance the robustness of your findings. Techniques like k-fold cross-validation allow you to partition your data into subsets, effectively minimizing the risk of overfitting while maximizing your model’s generalizability. This layered approach provides stronger confidence in the accuracy of your interpretations, reinforcing your overall methodological framework as you blend NLP with traditional polygraph practices.
Final Words
As a reminder, integrating Natural Language Processing (NLP) into polygraph examinations can significantly enhance your interpretation of results. By utilizing NLP technologies, you can analyze verbal cues, detect inconsistencies, and gain deeper insights into the subject’s emotional state. This integration not only streamlines your examination process but also enriches your understanding of the interplay between language and truthfulness. Embrace these innovative tools to improve your accuracy and effectiveness, ultimately fostering a more reliable assessment environment.
Q: How can Natural Language Processing (NLP) improve the accuracy of polygraph examinations?
A: Natural Language Processing can enhance the precision of polygraph examinations by analyzing the subject’s verbal responses in real-time. By employing algorithms that can detect linguistic cues, NLP can identify stress patterns, inconsistencies, and emotional states that may indicate truthfulness or deception. This analysis can supplement physiological data collected during examinations, such as heart rate and galvanic skin response, to provide a more comprehensive assessment of the subject’s credibility.
Q: What are some specific NLP techniques that can be used in polygraph examinations?
A: Several NLP techniques can be employed in polygraph examinations, including sentiment analysis, keyword extraction, and discourse analysis. Sentiment analysis can evaluate the emotional tone of the subject’s responses, helping to determine if their feelings align with their verbal statements. Keyword extraction can identify significant terms or phrases that may reveal patterns of truth or deception. Discourse analysis focuses on the structure and flow of the conversation, allowing examiners to detect abnormalities in language use that may require further investigation.
Q: What challenges might arise when integrating NLP into polygraph examinations?
A: Integrating NLP into polygraph examinations may present challenges such as the variability in human language, cultural nuances, and the need for high-quality training data. Language can be subjective and context-dependent, making it complex for NLP models to accurately interpret responses without misunderstanding the intent behind certain phrases. Additionally, ensuring that the NLP system is trained on diverse datasets that account for different dialects and cultural references is imperative for obtaining reliable results. Ongoing refinement and validation of the NLP tools will be necessary to address these challenges effectively.